AI-图像不变矩-图像识别的数学基础
2017-12-28 14:02
881 查看
AI基础-图像不变矩
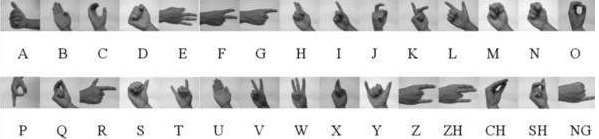
In image processing, computer
vision and related fields, an image moment is a certain particular weighted average (moment)
of the image pixels' intensities, or a function of such moments, usually chosen to have some attractive property or interpretation.
Image moments are useful to describe objects after segmentation. Simple
properties of the image which are found via image moments include area (or total intensity), its centroid,
and information about its orientation.
Mpq=∫−∞∞∫−∞∞xpyqf(x,y)dxdy

for p,q = 0,1,2,... Adapting this to scalar (greyscale) image with pixel intensities I(x,y), raw image moments Mij are calculated by
Mij=∑x∑yxiyjI(x,y)

In some cases, this may be calculated by considering the image as a probability
density function, i.e., by dividing the above by
∑x∑yI(x,y)

A uniqueness theorem (Hu [1962]) states that if f(x,y) is piecewise continuous and has nonzero values only in a finite part of the xy plane, moments of all orders exist, and the moment sequence
(Mpq) is uniquely determined by f(x,y). Conversely, (Mpq) uniquely determines f(x,y). In practice, the image is summarized
with functions of a few lower order moments.
Area (for binary images) or sum of grey level (for greytone images): M00
Centroid: { x, y } = {M10/M00, M01/M00 }
μpq=∫−∞∞∫−∞∞(x−x¯)p(y−y¯)qf(x,y)dxdy

where x¯=M10M00

and y¯=M01M00

are
the components of the centroid.
If ƒ(x, y) is a digital image, then the previous equation becomes
μpq=∑x∑y(x−x¯)p(y−y¯)qf(x,y)

The central moments of order up to 3 are:
μ00=M00,

μ01=0,

μ10=0,

μ11=M11−x¯M01=M11−y¯M10,

μ20=M20−x¯M10,

μ02=M02−y¯M01,

μ21=M21−2x¯M11−y¯M20+2x¯2M01,

μ12=M12−2y¯M11−x¯M02+2y¯2M10,

μ30=M30−3x¯M20+2x¯2M10,

μ03=M03−3y¯M02+2y¯2M01.

It can be shown that:
μpq=∑mp∑nq(pm)(qn)(−x¯)(p−m)(−y¯)(q−n)Mmn

Central moments are translational invariant.
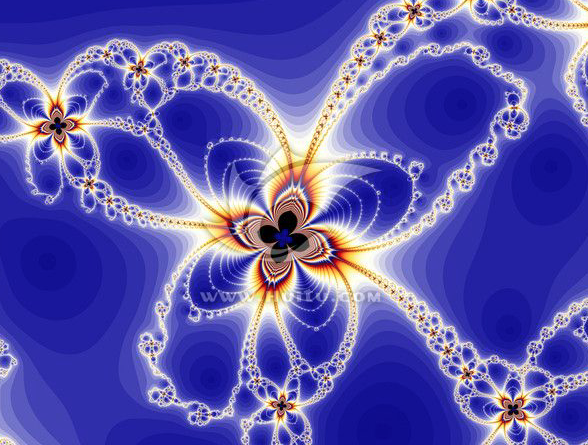
μ20′=μ20/μ00=M20/M00−x¯2

μ02′=μ02/μ00=M02/M00−y¯2

μ11′=μ11/μ00=M11/M00−x¯y¯

The covariance matrix of the image I(x,y)

is
now
cov[I(x,y)]=[μ20′μ11′μ11′μ02′]

.
The eigenvectors of this matrix correspond to the major and minor
axes of the image intensity, so the orientation can thus be extracted from the angle of the eigenvector associated with the largest eigenvalue towards the axis closest to this eigenvector. It can be shown that this angle Θ is given by the
following formula:
Θ=12arctan(2μ11′μ20′−μ02′)

The above formula holds as long as:
μ20′−μ02′≠0

The eigenvalues of the covariance matrix can easily be shown to be
λi=μ20′+μ02′2±4μ′112+(μ′20−μ′02)22,

and are proportional to the squared length of the eigenvector axes. The relative difference in magnitude of the eigenvalues are thus an indication of the eccentricity of the image, or how elongated it is. The eccentricity is
1−λ2λ1.

Moments are well-known for their application in image analysis, since they can be used to derive invariants with
respect to specific transformation classes.
The term invariant moments is often abused in this context. However, while moment invariants are invariants that are formed from moments, the only moments that are invariants themselves are the central moments.
Note that the invariants detailed below are exactly invariant only in the continuous domain. In a discrete domain, neither scaling nor rotation are well defined: a discrete image transformed in such a way is generally an approximation,
and the transformation is not reversible. These invariants therefore are only approximately invariant when describing a shape in a discrete image.
ηij=μijμ00(1+i+j2)

where i + j ≥ 2. Note that translational invariance directly follows by only using central moments.
I1=η20+η02

I2=(η20−η02)2+4η112

I3=(η30−3η12)2+(3η21−η03)2

I4=(η30+η12)2+(η21+η03)2

I5=(η30−3η12)(η30+η12)[(η30+η12)2−3(η21+η03)2]+(3η21−η03)(η21+η03)[3(η30+η12)2−(η21+η03)2]

I6=(η20−η02)[(η30+η12)2−(η21+η03)2]+4η11(η30+η12)(η21+η03)

I7=(3η21−η03)(η30+η12)[(η30+η12)2−3(η21+η03)2]−(η30−3η12)(η21+η03)[3(η30+η12)2−(η21+η03)2].

These are well-known as Hu moment invariants.
The first one, I1, is analogous to the moment
of inertia around the image's centroid, where the pixels' intensities are analogous to physical density. The last one, I7, is skew invariant, which enables it to distinguish mirror images of otherwise identical
images.
A general theory on deriving complete and independent sets of rotation moment invariants was proposed by J. Flusser. He showed that the traditional set of Hu moment invariants is not independent nor complete. I3 is
not very useful as it is dependent on the others. In the original Hu's set there is a missing third order independent moment invariant:
I8=η11[(η30+η12)2−(η03+η21)2]−(η20−η02)(η30+η12)(η03+η21)

Later, J. Flusser and T. Suk specialized the theory for N-rotationally symmetric shapes case.
In image processing, computer
vision and related fields, an image moment is a certain particular weighted average (moment)
of the image pixels' intensities, or a function of such moments, usually chosen to have some attractive property or interpretation.
Image moments are useful to describe objects after segmentation. Simple
properties of the image which are found via image moments include area (or total intensity), its centroid,
and information about its orientation.
Raw moments
For a 2D continuous function f(x,y)
the moment (sometimes
called "raw moment") of order (p + q)
is defined as
Mpq=∫−∞∞∫−∞∞xpyqf(x,y)dxdyfor p,q = 0,1,2,... Adapting this to scalar (greyscale) image with pixel intensities I(x,y), raw image moments Mij are calculated by
Mij=∑x∑yxiyjI(x,y)
In some cases, this may be calculated by considering the image as a probability
density function, i.e., by dividing the above by
∑x∑yI(x,y)
A uniqueness theorem (Hu [1962]) states that if f(x,y) is piecewise continuous and has nonzero values only in a finite part of the xy plane, moments of all orders exist, and the moment sequence
(Mpq) is uniquely determined by f(x,y). Conversely, (Mpq) uniquely determines f(x,y). In practice, the image is summarized
with functions of a few lower order moments.
Examples
Simple image properties derived via raw moments include:
Area (for binary images) or sum of grey level (for greytone images): M00Centroid: { x, y } = {M10/M00, M01/M00 }
Central moments
Central moments are
defined as
μpq=∫−∞∞∫−∞∞(x−x¯)p(y−y¯)qf(x,y)dxdywhere x¯=M10M00
and y¯=M01M00
are
the components of the centroid.
If ƒ(x, y) is a digital image, then the previous equation becomes
μpq=∑x∑y(x−x¯)p(y−y¯)qf(x,y)
The central moments of order up to 3 are:
μ00=M00,
μ01=0,
μ10=0,
μ11=M11−x¯M01=M11−y¯M10,
μ20=M20−x¯M10,
μ02=M02−y¯M01,
μ21=M21−2x¯M11−y¯M20+2x¯2M01,
μ12=M12−2y¯M11−x¯M02+2y¯2M10,
μ30=M30−3x¯M20+2x¯2M10,
μ03=M03−3y¯M02+2y¯2M01.
It can be shown that:
μpq=∑mp∑nq(pm)(qn)(−x¯)(p−m)(−y¯)(q−n)Mmn
Central moments are translational invariant.
Examples
Information about image orientation can be derived by first using the second order central moments to construct a covariance
matrix.
μ20′=μ20/μ00=M20/M00−x¯2μ02′=μ02/μ00=M02/M00−y¯2
μ11′=μ11/μ00=M11/M00−x¯y¯
The covariance matrix of the image I(x,y)
is
now
cov[I(x,y)]=[μ20′μ11′μ11′μ02′]
.
The eigenvectors of this matrix correspond to the major and minor
axes of the image intensity, so the orientation can thus be extracted from the angle of the eigenvector associated with the largest eigenvalue towards the axis closest to this eigenvector. It can be shown that this angle Θ is given by the
following formula:
Θ=12arctan(2μ11′μ20′−μ02′)
The above formula holds as long as:
μ20′−μ02′≠0
The eigenvalues of the covariance matrix can easily be shown to be
λi=μ20′+μ02′2±4μ′112+(μ′20−μ′02)22,
and are proportional to the squared length of the eigenvector axes. The relative difference in magnitude of the eigenvalues are thus an indication of the eccentricity of the image, or how elongated it is. The eccentricity is
1−λ2λ1.
Moment invariants
Moments are well-known for their application in image analysis, since they can be used to derive invariants withrespect to specific transformation classes.
The term invariant moments is often abused in this context. However, while moment invariants are invariants that are formed from moments, the only moments that are invariants themselves are the central moments.
Note that the invariants detailed below are exactly invariant only in the continuous domain. In a discrete domain, neither scaling nor rotation are well defined: a discrete image transformed in such a way is generally an approximation,
and the transformation is not reversible. These invariants therefore are only approximately invariant when describing a shape in a discrete image.
Translation invariants
The central moments μi j of any order are, by construction, invariant with respect to translations.
Scale invariants
Invariants ηi j with respect to both translation and scale can
be constructed from central moments by dividing through a properly scaled zero-th central moment:
ηij=μijμ00(1+i+j2)where i + j ≥ 2. Note that translational invariance directly follows by only using central moments.
Rotation invariants
As shown in the work of Hu et al., invariants with respect to translation, scale,
and rotation can be constructed:
I1=η20+η02I2=(η20−η02)2+4η112
I3=(η30−3η12)2+(3η21−η03)2
I4=(η30+η12)2+(η21+η03)2
I5=(η30−3η12)(η30+η12)[(η30+η12)2−3(η21+η03)2]+(3η21−η03)(η21+η03)[3(η30+η12)2−(η21+η03)2]
I6=(η20−η02)[(η30+η12)2−(η21+η03)2]+4η11(η30+η12)(η21+η03)
I7=(3η21−η03)(η30+η12)[(η30+η12)2−3(η21+η03)2]−(η30−3η12)(η21+η03)[3(η30+η12)2−(η21+η03)2].
These are well-known as Hu moment invariants.
The first one, I1, is analogous to the moment
of inertia around the image's centroid, where the pixels' intensities are analogous to physical density. The last one, I7, is skew invariant, which enables it to distinguish mirror images of otherwise identical
images.
A general theory on deriving complete and independent sets of rotation moment invariants was proposed by J. Flusser. He showed that the traditional set of Hu moment invariants is not independent nor complete. I3 is
not very useful as it is dependent on the others. In the original Hu's set there is a missing third order independent moment invariant:
I8=η11[(η30+η12)2−(η03+η21)2]−(η20−η02)(η30+η12)(η03+η21)
Later, J. Flusser and T. Suk specialized the theory for N-rotationally symmetric shapes case.
Hu moment invariants to solve the Pathological Brain Detection (PBD) problem.
相关文章推荐
- 从原理到代码:大牛教你如何用 TensorFlow 亲手搭建一套图像识别模块 | AI 研习社
- AI之图像识别
- 机器学习零基础?手把手教你用TensorFlow搭建图像识别系统(一)| 干货
- 如何在图片中加入噪点骗过Google最顶尖的图像识别AI
- [深度学习基础] 1. 图像识别问题的挑战及数据驱动过程
- AI:模式识别的数学表示(集合—函数观点)
- 游戏框架其九:3D图像之数学基础
- 从基础学AI---数学(1)
- PHP调用百度AI接口人脸识别-语音技术-文本识别-图像识别
- 向AI转行——人工智能工程师必学的数学基础
- 从原理到代码:大牛教你如何用 TensorFlow 亲手搭建一套图像识别模块 | AI 研习社
- 数字图像模式识别基础
- 【AI基础】python:openCV——图像算术运算(1)
- 机器学习零基础?手把手教你用TensorFlow搭建图像识别系统
- 图像处理与计算机视觉:基础,经典以及最近发展(3)计算机视觉中的信号处理与模式识别
- 数学之路(4)-数学基础-幂函数图像与函数平移
- [置顶] 百度ai—细粒度图像识别
- 图像识别算法基础
- AI数学基础(1)——贝叶斯公式
- AI钜惠第三弹 | 人工智能的数学基础