Hive基础
2017-10-31 00:00
85 查看
Hive架构简介
下图是hive的架构图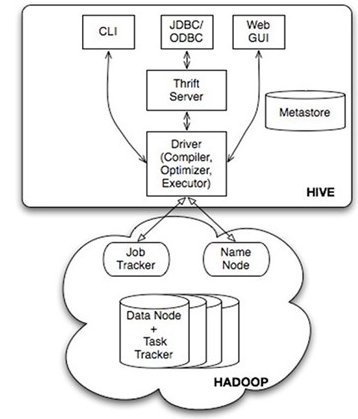
命令执行
所有的命令和查询都会进入到Driver,通过这个模块进行解析编译,对需求的计算进行优化。然后按照指定的步骤执行(通常是启动多个MapReduce任务(JOB)来执行)。当需要启动 MapReduce任务(job)时,HIVE 本身不会生成Java MapReduve算法程序。相反,Hive通过一个表示“JOB执行计划”的。XML文件驱动执行内置的、原生的Mapper和Reducer模块。换句话说,这些通用的模块函数类似于微型的语言翻译程序,二驱动计算的“语言”是以XML形式编码的。Hive 通过和JobTracker通信来初始化MapReduve任务(job),而不必部署在JobTracker所在的管理节点上执行。要处理的数据文件是存储在HDFS中的,而HDFS是由NameNode进行管理的。驱动器:Dirver 包含:解析器、编译器、优化器、执行器
解析器:将SQL字符串转换成抽象语法树AST,这一步一般都是用第三方工具库完成,比如antlr;对AST语 法树进行分析,比如表否存在、字段是否存在、SQL语义是否有误。
编译器:将AST编译生成逻辑执行计划。
优化器:对逻辑执行计划进行优化。
执行器:把逻辑执行计划转换成物理执行计划。对于hive来说,就是MR/TEZ/Spark;
元数据
Metastore是一个独立的关系型数据库,通常使用MYSQL。Hive 会在其中保存表模式和其他系统元数据中的。元数据包括表的名字,表的列和分区及其属性,表的属性(是否为外部表等),表的数据所在目录等Hive数据类型
hive基础数据类型
hive是用java开发的,hive里的基本数据类型和java的基本数据类型也是一一对应的,除了string类型。有符号的整数类型:TINYINT、SMALLINT、INT和BIGINT分别等价于java的byte、short、int和long原子类型,它们分别为1字节、2字节、4字节和8字节有符号整数。Hive的浮点数据类型FLOAT和DOUBLE,对应于java的基本类型float和double类型。而hive的BOOLEAN类型相当于java的基本数据类型boolean。hive复杂数据类型
包括ARRAY,MAP,STRUCT,UNION。这些复杂类型是由基础类型组成的。ARRAY:ARRAY类型是由一系列同样数据类型元素组成的,这些元素能够通过下标来訪问。比方有一个ARRAY类型的变量fruits。它是由[‘apple’,’orange’,’mango’]组成,那么能够通过fruits[1]来訪问orange。
MAP:MAP包括key->value键值对。能够通过key来訪问元素。比方userlist是一个map类型(当中username是key。password是value),那么我们能够通过userlist[username]来得到这个用户相应的password.。
STRUCT:STRUCT能够包括不同数据类型的元素。这些元素能够通过点的方式来得到,比方user是一个STRUCT类型,那么能够通过user.address得到这个用户的地址。
COLLECTION ITEMS TERMINATED BY ',' //集合元素分隔符
MAP KEYS TERMINATED BY ' : ' //MAP数据类型key:value分割符
Hive表类型
内部表内部表也称之为MANAGED_TABLE;默认存储在/user/hive/warehouse下,也可以通过location指定;删除表时,会删除表数据以及元数据;
create table if not exists...
外部表
外部表称之为EXTERNAL_TABLE在创建表时可以自己指定目录位置(LOCATION);删除表时,只会删除元数据不会删除表数据;
create EXTERNAL table if not exists...
加载数据
注意 导入的路径必须是hive用户有访问权限的路径,否则会报找不到文件错误
(1) HDFS上导入数据到Hive表
load data inpath '/home/wyp/add.txt' into table wyp;
(2) 从本地路径导入数据到Hive表
load data local inpath 'wyp.txt' into table wyp;
(3) 从别的表查询加载到hive表
静态分区
insert into table test partition (age='25') select id, name, tel from wyp;
动态分区
set hive.exec.dynamic.partition=true; set hive.exec.dynamic.partition.mode=nonstrict; insert overwrite table test PARTITION (age) select id, name, tel, age from wyp;
insert into table和insert overwrite table的区别:后者会覆盖相应数据目录下的数据将。
创建相似表
create table table_name like other_table_name location "xxxxx"
查询数据输出到本地目录
INSERT OVERWRITE DIRECTORY '/tmp/hdfs_out' SELECT a.* FROM invites a WHERE a.ds='<DATE>';
select *from emp distribute by deptno sort by empno asc ;
cluster by = distribute by 和 sort by 值相同。
Hive UDF编程
UDF(user defined function).hive的UDF包含三种:UDF支持一个输入产生一个输出,UDTF支持一个输入多个输出输出(一行变多行),UDAF支持多输入一输出(多行变一行)。UDF 简单实现
编程步骤:1、继承org.apache.hadoop.hive.ql.UDF
2、需要实现evaluate函数; evaluate函数支持重载;
注意事项:
1、 UDF必须要有返回类型,可以返回null,但是返回类型不能为void;
2、 UDF中常用Text/LongWritable等类型,不推荐使用java类型;
官网demo: https://cwiki.apache.org/confluence/display/Hive/HivePlugins
使用UDF非常简单,只需要继承org.apache.hadoop.hive.ql.exec.UDF,并定义public Object evaluate(Object args) {} 方法即可。
比如,下面的UDF函数实现了对一个String类型的字符串取HashMD5:
package com.lxw1234.hive.udf; import org.apache.hadoop.hbase.util.Bytes; import org.apache.hadoop.hbase.util.MD5Hash; import org.apache.hadoop.hive.ql.exec.UDF; public class HashMd5 extends UDF { public String evaluate(String cookie) { return MD5Hash.getMD5AsHex(Bytes.toBytes(cookie)); } }
将上面的HashMd5类打成jar包,udf.jar
使用时候,在Hive命令行执行:
hive> add jar file:///tmp/udf.jar; hive> CREATE temporary function str_md5 as 'com.lxw1234.hive.udf.HashMd5'; hive> select str_md5(‘lxw1234.com’) from dual;
Transform
Hive中的TRANSFORM:使用脚本完成Map/Reduce。hive> add file python文件路径 hive> SELECT TRANSFORM(p.joint_attr_values, p.collect_product_id, p.released_id) USING 'python split_product_attrs.py' as (custom_attr , custom_attr_value, collect_product_id, released_product_id) from ( 这里应该是另外一个select语句,用于Transform的输入,最好是一一对应的,否则会出错 )
注意:python脚本一定要先加载:hive>ADD FILE python_file_path [注意此处的path不要加''上引号]
下面是python的脚本,用于将三列转换为四列,这里就比较简单了,主要用于测试,代码随便写了一下
#!/usr/bin/python # #_*_ coding: utf-8 _*_ import sys import datetime # "规格:RN1-10/50;规格:RN1-10/50;规格:RN1-10/50" # ["规格:RN1-10/51;规格:RN1-10/52;规格:RN1-10/53", '11', '22'] # ["规格", "RN1-10/51", '11', '22'] # ["规格", "RN1-10/52", '11', '22'] # ["规格", "RN1-10/53", '11', '22'] for line in sys.stdin: values = line.split('\t') values = [ i.strip() for i in values ] tmp = values[0] key_values = tmp.split(";") for kv in key_values: k = kv.split(":")[0] v = kv.split(":")[1] print '\t'.join([k,v,values[1],values[2]])
Hive企业优化方案
Hive存储格式
1、TEXTFILE 默认格式,建表时不指定默认为这个格式,导入数据时会直接把数据文件拷贝到hdfs上不进行处理。只有TEXTFILE表能直接加载数据,本地load数据,和external外部表直接加载运路径数据,都只能用TEXTFILE表。更深一步,hive默认支持的压缩文件(hadoop默认支持的压缩格式),也只能用TEXTFILE表直接读取。其他格式不行。可以通过TEXTFILE表加载后insert到其他表中。2、orc 格式。 STORED AS ORC ;
3、parquet 格式。 STORED AS PARQUET ;
几种格式的差别 http://www.cnblogs.com/juncaoit/p/6067646.html
Hive压缩优化
hive压缩相关博客 https://yq.aliyun.com/articles/60859压缩配置:
map/reduce 输出压缩(一般采用序列化文件存储)
set hive.exec.compress.output=true; set mapred.output.compression.codec=org.apache.hadoop.io.compress.GzipCodec; set mapred.output.compression.type=BLOCK;
任务中间压缩
set hive.exec.compress.intermediate=true; set hive.intermediate.compression.codec=org.apache.hadoop.io.compress.SnappyCodec;(常用) set hive.intermediate.compression.type=BLOCK;
中间压缩
中间压缩就是处理作业map任务和reduce任务之间的数据,对于中间压缩,最好选择一个节省CPU耗时的压缩方式
<property> <name>hive.exec.compress.intermediate</name> <value>true</value> </property>
hadoop压缩有一个默认的压缩格式,当然可以通过修改mapred.map.output.compression.codec属性,使用新的压缩格式,这个变量可以在mapred-site.xml 中设置或者在 hive-site.xml文件。 SnappyCodec 是一个较好的压缩格式,CPU消耗较低。
<property> <name>mapred.map.output.compression.codec</name> <value>org.apache.hadoop.io.compress.SnappyCodec</value> </property>
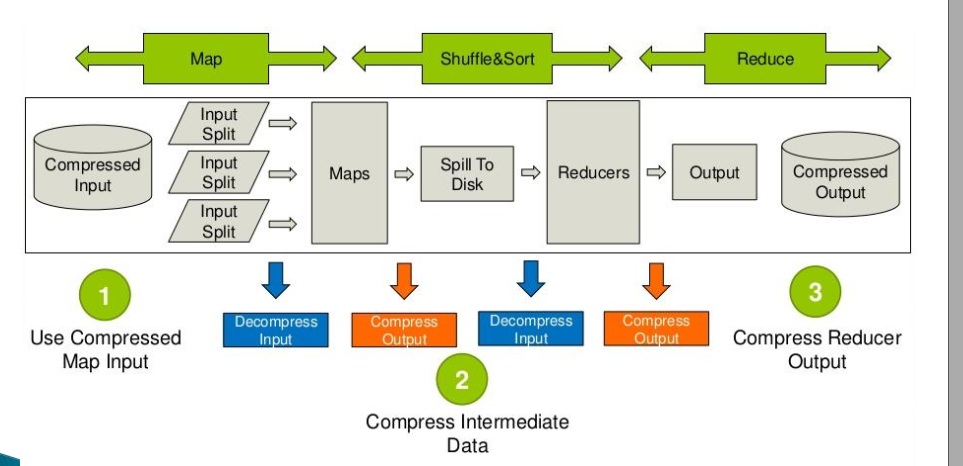
MapReduce中使用压缩
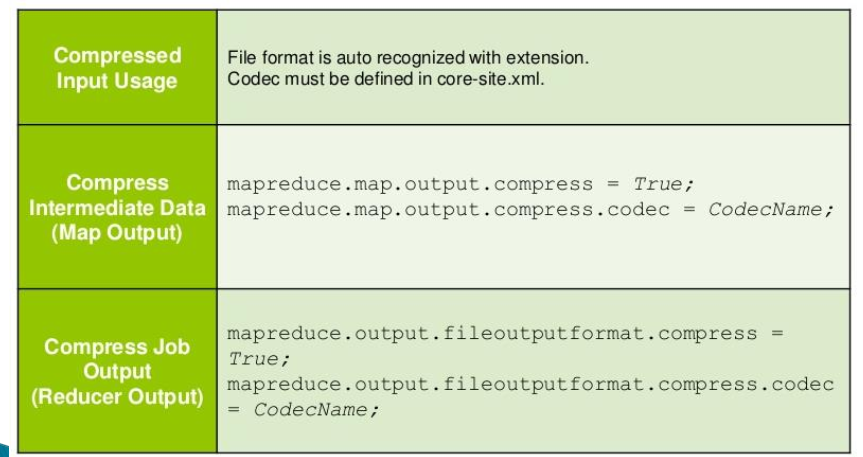
Hive中 使用压缩
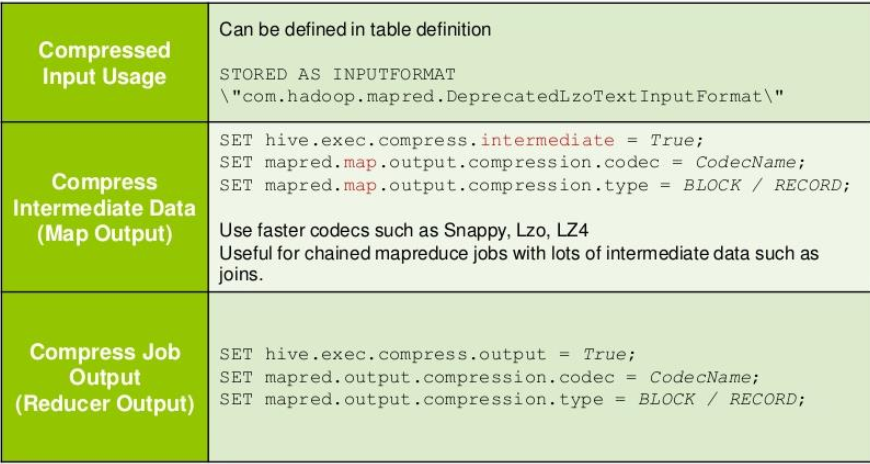
关闭推测执行
(1) 修改mapred-site.xmlmapreduce.map.speculative false
mapreduce.reduce.speculativ false
(2) 设置值 set hive.mapred.reduce.tasks.speculative.execution=true;
Hive 数据倾斜解决方案
Hive join解释 https://www.cnblogs.com/xing901022/p/5804836.html小表 join 大表 大表 join 大表 方案
(1)Common/Shuffle/Reduce Join
普通mapreduce join ,相同key分配到同一个reducer。(2) Map Join
MAPJION会把小表全部读入内存中,在map阶段直接拿另外一个表的数据和内存中表数据做匹配,适用于小表join 大表。set hive.auto.convert.join=false; set hive.ignore.mapjoin.hint=false;
Sql可以通过使用hint的方式指定join时使用mapjoin,其中mapjoin选中的表为小表
select /*+ mapjoin(t1)*/ t1.a,t1.b from table t1 join table2 t2 on ( t1.a=t2.a and t1.time=201108)
Map Join Java实现 http://www.cnblogs.com/ivanny/p/mapreduce_join.html
(3) SMB Join (Sort Merge Bucket Join)
使用SMB两个表必须使用桶分区,使用 SMB Join 需要如下配置set hive.enforce.bucketing = true; //强制使用桶表 set hive.auto.convert.sortmerge.join=true; set hive.optimize.bucketmapjoin = true; set hive.optimize.bucketmapjoin.sortedmerge = true;
select /*+ mapjoin(t1)*/ t1.a,t1.b from table t1 join table2 t2 on ( t1.a=t2.a and t1.time=201108)
1. 两个表关联键为id,需要按id分桶并且做排序,小表的分桶数是大表分桶数的倍数。
2. 对于map端连接的情况,两个表以相同方式划分桶。处理左边表内某个桶的 mapper知道右边表内相匹配的行在对应的桶内。因此,mapper只需要获取那个桶 (这只是右边表内存储数据的一小部分)即可进行连接。这一优化方法并不一定要求 两个表必须桶的个数相同,两个表的桶个数是倍数关系也可以。
3. 桶中的数据可以根据一个或多个列另外进行排序。由于这样对每个桶的连接变成了高效的归并排序(merge-sort), 因此可以进一步提升map端连接的效率。
Hive分区
建表create table user_info (user_id int, cid string, ckid string, username string) row format delimited fields terminated by '\t' lines terminated by '\n'; 1.行格式化 2.字段通过'\t' 分割 3.行通过'\n' 分隔开
# flume 收集 建表方案
create table baoertest(user_agent string,user_id bigint,time string) partitioned by (year string, month string, day string) clustered by (user_id) into 5 buckets stored as orc tblproperties("transactional"="true"); #使用这个,不能使用insert overwrite,可以使用insert,delete
# insert overwrite table 建表方案 prestodb可识别
create table baoertest(user_agent string,user_id bigint,time string) partitioned by (year string, month string, day string) clustered by (user_id) into 5 buckets stored as orc;
set hive.enforce.bucketing=true; # 使用桶,需要强制设置
set mapreduce.job.reduces=<number> # 设置reduces个数
#静态分区
create table baoertest_txt(user_agent string,user_id bigint,time string) ROW FORMAT DELIMITED FIELDS TERMINATED BY '\t'; LOAD DATA LOCAL INPATH '/data/hive/data.txt' OVERWRITE INTO TABLE baoertest_txt; INSERT OVERWRITE TABLE baoertest PARTITION (year="2015",month="10",day="12") select * from baoertest_txt;
--data.txt--
chrome 112212123 11-12
windows 123123123 11-11
#动态分区
set hive.exec.dynamic.partition=true; #动态分区 set hive.exec.dynamic.partition.mode=nonstrict; #动态分区非严格模式 create table baoertest_txt01(user_agent string,user_id bigint,time string,year string, month string, day string) ROW FORMAT DELIMITED FIELDS TERMINATED BY '\t'; LOAD DATA LOCAL INPATH '/data/hive/data01.txt' OVERWRITE INTO TABLE baoertest_txt01; INSERT OVERWRITE TABLE baoertest PARTITION (year,month,day) select * from baoertest_txt01;
--data01.txt--
chrome 112212123 11-12 2017 05 21
windows 123123123 11-11 2017 06 19
#查询使用分区
当分区建立后,查询可直接使用分区字段
select *from baoertest where year=xxx and month=xxx and day=xxx
#手动添加分区
alter table table_xxx add partition (dt='2016-09-12');
#手动删除分区
alter table table_xxx drop partition (month=2017,month=10,day=21);
Hive ACID事务配置
hive-site.xml
<!-- hive acid 事务支持 --> <property> <name>hive.support.concurrency</name> <value>true</value> </property> <property> <name>hive.txn.manager</name> <value>org.apache.hadoop.hive.ql.lockmgr.DbTxnManager</value> </property> <property> <name>hive.enforce.bucketing</name> <value>true</value> </property> <property> <name>hive.compactor.initiator.on</name> <value>true</value> </property> <property> <name>hive.compactor.worker.threads</name> <value>2</value> </property> <property> <name>hive.exec.dynamic.partition.mode</name> <value>nonstrict</value> </property> <property> <name>hive.compactor.initiator.on</name> <value>true</value> </property> <property> <name>hive.in.test</name> <value>true</value> </property>
Hive SQL验证acid特性
create table table2_test ( EmployeeID Int, FirstName String, Designation String, Salary Int,Department String ) clustered by (department) into 3 buckets stored as orc TBLPROPERTIES ('transactional'='true'); Insert into table table2_test values (102, 'Employee102','Designation102', 10101, 'Dept102'), (103, 'Employee103','Designation103', 10102, 'Dept103'); update table2_test set firstname='gaojunxiu111' where salary=10101;
相关文章推荐
- Hadoop生态之Awesome系列
- 分享Hive的一份胶片资料
- MySQL 优化
- Hadoop生态上几个技术的关系与区别:hive、pig、hbase 关系与区别
- 5分钟了解MySQL5.7对in用法有什么黑科技
- Google排名优化的几个影响因素
- DB2优化(简易版)
- Mysql limit 优化,百万至千万级快速分页 复合索引的引用并应用于轻量级框架
- C#中尾递归的使用、优化及编译器优化
- 详解vue项目首页加载速度优化
- 对优化Ruby on Rails性能的一些办法的探究
- 优化Ruby脚本效率实例分享
- Redis优化经验总结(必看篇)
- Asp编码优化技巧
- SQLServer性能优化--间接实现函数索引或者Hash索引
- 如何监测和优化OLAP数据库
- mysql -参数thread_cache_size优化方法 小结
- MySQL数据库优化技术之配置技巧总结
- Oracle数据库中SQL语句的优化技巧
- 深入学习SQL Server聚合函数算法优化技巧