(原)Matlab的svmtrain和svmclassify
2016-06-02 22:00
429 查看
转载请注明出处:
http://www.cnblogs.com/darkknightzh/p/5554551.html
参考网址:
http://www.cnblogs.com/zhangchaoyang/articles/2222048.html
代码如下:
分类结果:
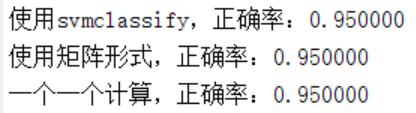
svmtrain后的结果(包含了svmclassify的结果),其中蓝色圈出来的点为分错的点。
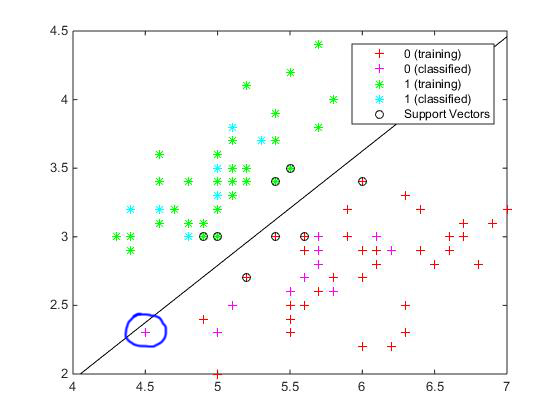
实际上,如果4个特征都使用的话,对上面的程序,正确率为100%。
ps:测试数据为参考网址中给出的数据。
鹫尾花数据集共分为3类花(前50个样本为一类,中间50个样本为一类,后50个样本为一类。由于matlab的svmtrain只能处理二分类问题,因而程序中使用了前100个数据。其中,每组前40个用作训练,后10个用作测试。因而,训练样本80个,测试样本20个。除此之外,为了在调用svmtrain时能显示分类结果,该函数参数'showplot'设置为true。
data.txt如下:
http://www.cnblogs.com/darkknightzh/p/5554551.html
参考网址:
http://www.cnblogs.com/zhangchaoyang/articles/2222048.html
代码如下:
clc clear all close all data = load('data.txt'); % 为了svmtrain能使用'showplot',true,此处使用数据的前2维。 data = data(:, 1:2); % 由于svmtrain只能处理二分类问题,因而此处使用前100个数据。 data = data(1:100, :); label = [ones(50, 1); zeros(50, 1)]; trainData = data(1:40, :); % 每组前40个用于训练 trainData = [trainData; data(51:90, :)]; % 每组前40个用于训练 testData = data(41:50, :); % 每组后10个用于测试 testData = [testData; data(91:100, :)]; % 每组后10个用于测试 trainLabel = label(1:40, :); % 每组前40个用于训练 trainLabel = [trainLabel; label(51:90, :)]; % 每组前40个用于训练 testLabel = label(41:50, :); % 每组后10个用于测试 testLabel = [testLabel; label(91:100, :)]; % 每组后10个用于测试 % 将距离和类别传入svm中,trainLabel为标签,即类别,trainData为特征 svmModel = svmtrain(trainData, trainLabel,'kernel_function','linear','showplot',true); predict_label = svmclassify(svmModel,testData,'showplot',true); fprintf('使用svmclassify,正确率:%f\n' ,sum(predict_label==testLabel)/size(testLabel,1)); %% 以下两种方式均使用linear核,其他的核还不清楚怎么算 testScale = []; for c = 1:size(testData, 2) testScale = [testScale, svmModel.ScaleData.scaleFactor(c) * (testData(:,c) + svmModel.ScaleData.shift(c))]; end % 方式1:使用矩阵形式 predictValMat = (svmModel.SupportVectors * testScale')' * svmModel.Alpha + svmModel.Bias; % 测试样本个数*1的列矩阵 predictValMat1 = ones(size(predictValMat)); predictValMat1(predictValMat>0) = 0; fprintf('使用矩阵形式,正确率:%f\n' ,sum(predictValMat1==testLabel)/size(testLabel,1)); % 方式2:一个一个计算 [m,n]=size(testScale); predict_label2=zeros(m,1); for k = 1:size(testScale, 1) % svmModel.SupportVectors为 支持向量个数*特征维数 的矩阵 % testScale(k, :)为 1*特征维数 的行向量。 % svmModel.SupportVectors * testScale(k, :)'为 支持向量个数*1 的列矩阵 % (svmModel.SupportVectors * testScale(k, :)')' * svmModel.Alpha 即为 sum(w*x) % predictVal为 sum(w*x)+b predictVal = (svmModel.SupportVectors * testScale(k, :)')' * svmModel.Alpha + svmModel.Bias; if predictVal>0 predict_label2(k) = 0; else predict_label2(k) = 1; end end fprintf('一个一个计算,正确率:%f\n' ,sum(predict_label2==testLabel)/size(testLabel,1));
分类结果:
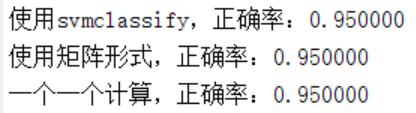
svmtrain后的结果(包含了svmclassify的结果),其中蓝色圈出来的点为分错的点。
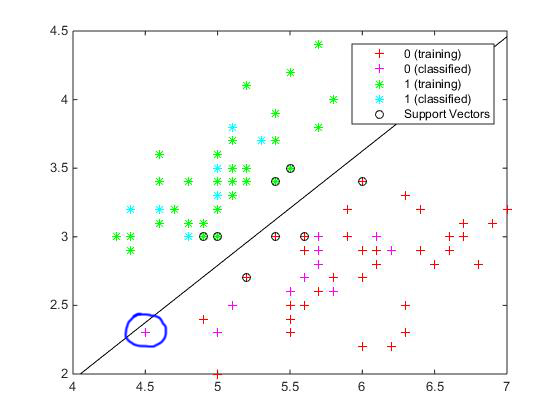
实际上,如果4个特征都使用的话,对上面的程序,正确率为100%。
ps:测试数据为参考网址中给出的数据。
鹫尾花数据集共分为3类花(前50个样本为一类,中间50个样本为一类,后50个样本为一类。由于matlab的svmtrain只能处理二分类问题,因而程序中使用了前100个数据。其中,每组前40个用作训练,后10个用作测试。因而,训练样本80个,测试样本20个。除此之外,为了在调用svmtrain时能显示分类结果,该函数参数'showplot'设置为true。
data.txt如下:
5.1 3.5 1.4 0.2 4.9 3.0 1.4 0.2 4.7 3.2 1.3 0.2 4.6 3.1 1.5 0.2 5.0 3.6 1.4 0.2 5.4 3.9 1.7 0.4 4.6 3.4 1.4 0.3 5.0 3.4 1.5 0.2 4.4 2.9 1.4 0.2 4.9 3.1 1.5 0.1 5.4 3.7 1.5 0.2 4.8 3.4 1.6 0.2 4.8 3.0 1.4 0.1 4.3 3.0 1.1 0.1 5.8 4.0 1.2 0.2 5.7 4.4 1.5 0.4 5.4 3.9 1.3 0.4 5.1 3.5 1.4 0.3 5.7 3.8 1.7 0.3 5.1 3.8 1.5 0.3 5.4 3.4 1.7 0.2 5.1 3.7 1.5 0.4 4.6 3.6 1.0 0.2 5.1 3.3 1.7 0.5 4.8 3.4 1.9 0.2 5.0 3.0 1.6 0.2 5.0 3.4 1.6 0.4 5.2 3.5 1.5 0.2 5.2 3.4 1.4 0.2 4.7 3.2 1.6 0.2 4.8 3.1 1.6 0.2 5.4 3.4 1.5 0.4 5.2 4.1 1.5 0.1 5.5 4.2 1.4 0.2 4.9 3.1 1.5 0.1 5.0 3.2 1.2 0.2 5.5 3.5 1.3 0.2 4.9 3.1 1.5 0.1 4.4 3.0 1.3 0.2 5.1 3.4 1.5 0.2 5.0 3.5 1.3 0.3 4.5 2.3 1.3 0.3 4.4 3.2 1.3 0.2 5.0 3.5 1.6 0.6 5.1 3.8 1.9 0.4 4.8 3.0 1.4 0.3 5.1 3.8 1.6 0.2 4.6 3.2 1.4 0.2 5.3 3.7 1.5 0.2 5.0 3.3 1.4 0.2 7.0 3.2 4.7 1.4 6.4 3.2 4.5 1.5 6.9 3.1 4.9 1.5 5.5 2.3 4.0 1.3 6.5 2.8 4.6 1.5 5.7 2.8 4.5 1.3 6.3 3.3 4.7 1.6 4.9 2.4 3.3 1.0 6.6 2.9 4.6 1.3 5.2 2.7 3.9 1.4 5.0 2.0 3.5 1.0 5.9 3.0 4.2 1.5 6.0 2.2 4.0 1.0 6.1 2.9 4.7 1.4 5.6 2.9 3.6 1.3 6.7 3.1 4.4 1.4 5.6 3.0 4.5 1.5 5.8 2.7 4.1 1.0 6.2 2.2 4.5 1.5 5.6 2.5 3.9 1.1 5.9 3.2 4.8 1.8 6.1 2.8 4.0 1.3 6.3 2.5 4.9 1.5 6.1 2.8 4.7 1.2 6.4 2.9 4.3 1.3 6.6 3.0 4.4 1.4 6.8 2.8 4.8 1.4 6.7 3.0 5.0 1.7 6.0 2.9 4.5 1.5 5.7 2.6 3.5 1.0 5.5 2.4 3.8 1.1 5.5 2.4 3.7 1.0 5.8 2.7 3.9 1.2 6.0 2.7 5.1 1.6 5.4 3.0 4.5 1.5 6.0 3.4 4.5 1.6 6.7 3.1 4.7 1.5 6.3 2.3 4.4 1.3 5.6 3.0 4.1 1.3 5.5 2.5 4.0 1.3 5.5 2.6 4.4 1.2 6.1 3.0 4.6 1.4 5.8 2.6 4.0 1.2 5.0 2.3 3.3 1.0 5.6 2.7 4.2 1.3 5.7 3.0 4.2 1.2 5.7 2.9 4.2 1.3 6.2 2.9 4.3 1.3 5.1 2.5 3.0 1.1 5.7 2.8 4.1 1.3 6.3 3.3 6.0 2.5 5.8 2.7 5.1 1.9 7.1 3.0 5.9 2.1 6.3 2.9 5.6 1.8 6.5 3.0 5.8 2.2 7.6 3.0 6.6 2.1 4.9 2.5 4.5 1.7 7.3 2.9 6.3 1.8 6.7 2.5 5.8 1.8 7.2 3.6 6.1 2.5 6.5 3.2 5.1 2.0 6.4 2.7 5.3 1.9 6.8 3.0 5.5 2.1 5.7 2.5 5.0 2.0 5.8 2.8 5.1 2.4 6.4 3.2 5.3 2.3 6.5 3.0 5.5 1.8 7.7 3.8 6.7 2.2 7.7 2.6 6.9 2.3 6.0 2.2 5.0 1.5 6.9 3.2 5.7 2.3 5.6 2.8 4.9 2.0 7.7 2.8 6.7 2.0 6.3 2.7 4.9 1.8 6.7 3.3 5.7 2.1 7.2 3.2 6.0 1.8 6.2 2.8 4.8 1.8 6.1 3.0 4.9 1.8 6.4 2.8 5.6 2.1 7.2 3.0 5.8 1.6 7.4 2.8 6.1 1.9 7.9 3.8 6.4 2.0 6.4 2.8 5.6 2.2 6.3 2.8 5.1 1.5 6.1 2.6 5.6 1.4 7.7 3.0 6.1 2.3 6.3 3.4 5.6 2.4 6.4 3.1 5.5 1.8 6.0 3.0 4.8 1.8 6.9 3.1 5.4 2.1 6.7 3.1 5.6 2.4 6.9 3.1 5.1 2.3 5.8 2.7 5.1 1.9 6.8 3.2 5.9 2.3 6.7 3.3 5.7 2.5 6.7 3.0 5.2 2.3 6.3 2.5 5.0 1.9 6.5 3.0 5.2 2.0 6.2 3.4 5.4 2.3 5.9 3.0 5.1 1.8
相关文章推荐
- matlab画频率直方图,支持颜色变换
- matlab 简单的人脸识别
- ubuntu 装matlab
- matlab 图像保存
- 函数句柄matlab
- Matlab将矩阵保存为图像
- 深度学习系列文章之四:ubantu系统下MATLAB安装
- 最近邻(NNC)
- 【matlab函数】——str2func函数的使用
- MATLAB基础之矩阵相关的建立、基本操作
- 关于MATLAB中imfilter函数的说明
- Matlab 图像处理 形态学 腐蚀 膨胀 开闭运算 连通分量
- [Matlab] matlab与C/C++混合编程汇总
- Matlab生成棋盘格点图
- Windows下Faster R-CNN 配置/Matlab版本编译
- K-近邻法(K-NN)
- Matlab 画柱状图
- 第一次打开matlab
- [Matlab] 如何将axes填充整个Figure
- matlab错误:Subscript indices must either be real positive integers or logicals.