memcache的一致性hash算法使用
2016-03-29 11:49
435 查看
原文:http://blog.csdn.net/kongqz/article/details/6695417
一、概述
1、什么是spymemcached
spymemcached是一个memcached的客户端,http://code.google.com/p/spymemcached/
它使用了一致性hash算法ketama进行数据存储节点的选择。与常规的hash算法思路不同,只是对我们要存储数据的key进行hash计算,分配到不同节点存储。一致性hash算法是对我们要存储数据的服务器进行hash计算,进而确认每个key的存储位置。
2、常规hash算法的应用以及其弊端
最常规的方式莫过于hash取模的方式。比如集群中可用机器适量为N,那么key值为K的的数据请求很简单的应该路由到hash(K) mod N对应的机器。的确,这种结构是简单的,也是实用的。但是在一些高速发展的web系统中,这样的解决方案仍有些缺陷。随着系统访问压力的增长,缓存系统不得不通过增加机器节点的方式提高集群的相应速度和数据承载量。增加机器意味着按照hash取模的方式,在增加机器节点的这一时刻,大量的缓存命不中,缓存数据需要重新建立,甚至是进行整体的缓存数据迁移,瞬间会给DB带来极高的系统负载,设置导致DB服务器宕机。
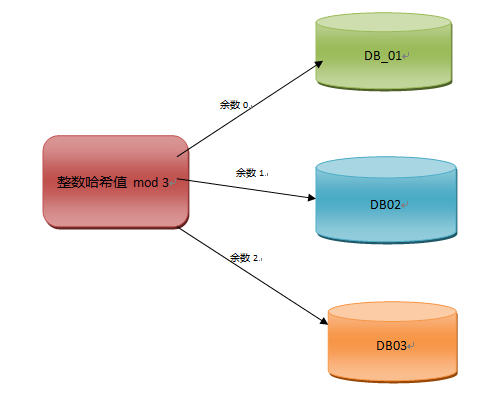
3、设计分布式cache系统时,一致性hash算法可以帮我们解决哪些问题?
分布式缓存设计核心点:在设计分布式cache系统的时候,我们需要让key的分布均衡,并且在增加cache server后,cache的迁移做到最少。
这里提到的一致性hash算法ketama的做法是:选择具体的机器节点不在只依赖需要缓存数据的key的hash本身了,而是机器节点本身也进行了hash运算。
二、一致性哈希算法情景描述
1、 hash机器节点
首先求出机器节点的hash值(怎么算机器节点的hash?ip可以作为hash的参数吧。。当然还有其他的方法了),然后将其分布到0~2^32的一个圆环上(顺时针分布)。如下图所示:
图1-1
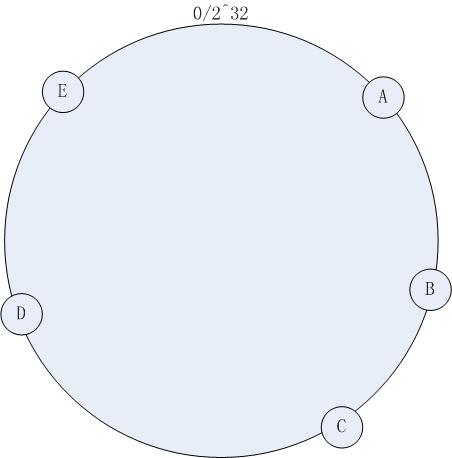
图1-2
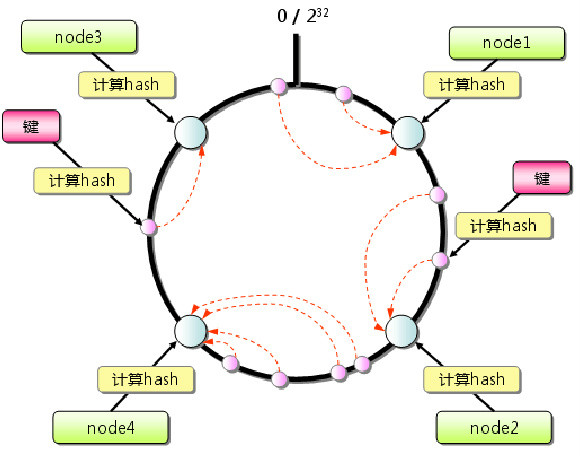
集群中有机器:A , B, C, D, E五台机器,通过一定的hash算法我们将其分布到如上图所示的环上。
2、访问方式
如果有一个写入缓存的请求,其中Key值为K,计算器hash值Hash(K), Hash(K) 对应于图 – 1环中的某一个点,如果该点对应没有映射到具体的某一个机器节点,那么顺时针查找,直到第一次找到有映射机器的节点,该节点就是确定的目标节点,如果超过了2^32仍然找不到节点,则命中第一个机器节点。比如 Hash(K) 的值介于A~B之间,那么命中的机器节点应该是B节点(如上图 )。
3、增加节点的处理
如上图 1– 1和图1-2,在原有集群的基础上欲增加一台机器F,增加过程如下:
计算机器节点的Hash值,将机器映射到环中的一个节点,如下图:
图2-1
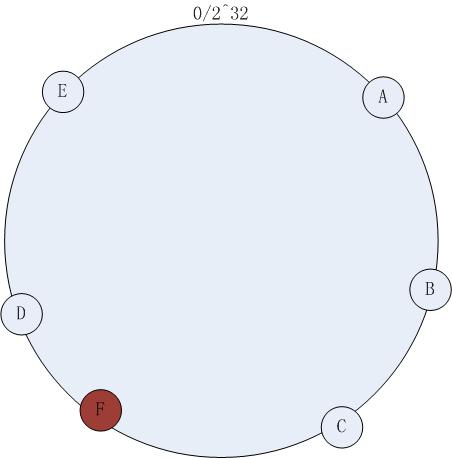
图2-2
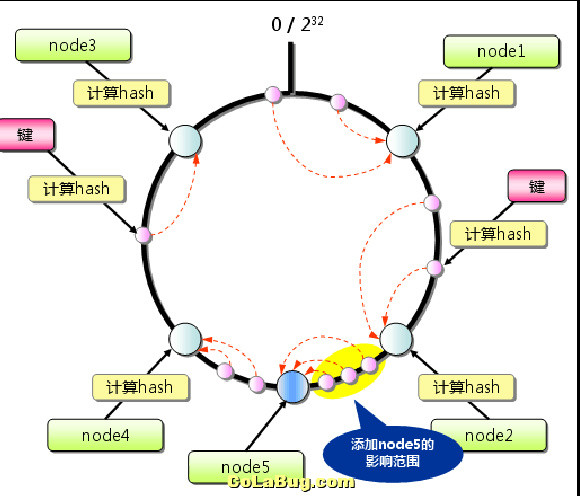
增加机器节点F之后,访问策略不改变,依然按照(2)中的方式访问,此时缓存命不中的情况依然不可避免,不能命中的数据是hash(K)在增加节点以前落在C~F之间的数据。尽管依然存在节点增加带来的命中问题,但是比较传统的 hash取模的方式,一致性hash已经将不命中的数据降到了最低。
Consistent Hashing最大限度地抑制了hash键的重新分布。另外要取得比较好的负载均衡的效果,往往在服务器数量比较少的时候需要增加虚拟节点来保证服务器能均匀的分布在圆环上。因为使用一般的hash方法,服务器的映射地点的分布非常不均匀。使用虚拟节点的思想,为每个物理节点(服务器)在圆上分配100~200个点。这样就能抑制分布不均匀,最大限度地减小服务器增减时的缓存重新分布。用户数据映射在虚拟节点上,就表示用户数据真正存储位置是在该虚拟节点代表的实际物理服务器上。
下面有一个图描述了需要为每台物理服务器增加的虚拟节点。

图三
x轴表示的是需要为每台物理服务器扩展的虚拟节点倍数(scale),y轴是实际物理服务器数,可以看出,当物理服务器的数量很小时,需要更大的虚拟节点,反之则需要更少的节点,从图上可以看出,在物理服务器有10台时,差不多需要为每台服务器增加100~200个虚拟节点才能达到真正的负载均衡。
三、以spymemcache源码来演示虚拟节点应用
1、上边描述的一致性Hash算法有个潜在的问题是:
(1)、将节点hash后会不均匀地分布在环上,这样大量key在寻找节点时,会存在key命中各个节点的概率差别较大,无法实现有效的负载均衡。
(2)、如有三个节点Node1,Node2,Node3,分布在环上时三个节点挨的很近,落在环上的key寻找节点时,大量key顺时针总是分配给Node2,而其它两个节点被找到的概率都会很小。
2、这种问题的解决方案可以有:
改善Hash算法,均匀分配各节点到环上;[引文]使用虚拟节点的思想,为每个物理节点(服务器)在圆上分配100~200个点。这样就能抑制分布不均匀,最大限度地减小服务器增减时的缓存重新分布。用户数据映射在虚拟节点上,就表示用户数据真正存储位置是在该虚拟节点代表的实际物理服务器上。
在查看Spy Memcached client时,发现它采用一种称为Ketama的Hash算法,以虚拟节点的思想,解决Memcached的分布式问题。
3、源码说明
该client采用TreeMap存储所有节点,模拟一个环形的逻辑关系。在这个环中,节点之前是存在顺序关系的,所以TreeMap的key必须实现Comparator接口。
那节点是怎样放入这个环中的呢?
KetamaNodeLocator.java中
[html] view
plain copy
protected void setKetamaNodes(List<MemcachedNode> nodes) {
TreeMap<Long, MemcachedNode> newNodeMap = new TreeMap<Long, MemcachedNode>();
int numReps= config.getNodeRepetitions();
for(MemcachedNode node : nodes) {
// Ketama does some special work with md5 where it reuses chunks.
if(hashAlg == HashAlgorithm.KETAMA_HASH) {
for(int i=0; i<numReps / 4; i++) {
byte[] digest=HashAlgorithm.computeMd5(config.getKeyForNode(node, i));
for(int h=0;h<4;h++) {
Long k = ((long)(digest[3+h*4]&0xFF) << 24)
| ((long)(digest[2+h*4]&0xFF) << 16)
| ((long)(digest[1+h*4]&0xFF) << 8)
| (digest[h*4]&0xFF);
newNodeMap.put(k, node);
getLogger().debug("Adding node %s in position %d", node, k);
}
}
} else {
for(int i=0; i<numReps; i++) {
newNodeMap.put(hashAlg.hash(config.getKeyForNode(node, i)), node);
}
}
}
assert newNodeMap.size() == numReps * nodes.size();
ketamaNodes = newNodeMap;
config.getNodeRepetitions()表示的是需要为每台物理服务器扩展的虚拟节点倍数(scale)。
上面的流程大概可以这样归纳:四个虚拟结点为一组,以getKeyForNode方法得到这组虚拟节点的name,Md5编码后,每个虚拟结点对应Md5码16个字节中的4个,组成一个long型数值,做为这个虚拟结点在环中的惟一key。第10行k为什么是Long型的呢?就是因为Long型实现了Comparator接口。
默认节点配置文件如下:DefaultKetamaNodeLocatorConfiguration.java
处理完正式结点在环上的分布后,可以开始key在环上寻找节点的游戏了。
对于每个key还是得完成上面的步骤:计算出Md5,根据Md5的字节数组,通过Kemata Hash算法得到key在这个环中的位置。
KetamaNodeLocator.java中
[html] view
plain copy
MemcachedNode getNodeForKey(long hash) {
final MemcachedNode rv;
if(!ketamaNodes.containsKey(hash)) {
// Java 1.6 adds a ceilingKey method, but I'm still stuck in 1.5
// in a lot of places, so I'm doing this myself.
SortedMap<Long, MemcachedNode> tailMap=getKetamaNodes().tailMap(hash);
if(tailMap.isEmpty()) {
hash=getKetamaNodes().firstKey();
} else {
hash=tailMap.firstKey();
}
}
rv=getKetamaNodes().get(hash);
return rv;
}
上边代码的实现就是在环上顺时针查找,没找到就去的第一个,然后就知道对应的物理节点了。
四、应用场景分析
1、memcache的add方法:通过一致性hash算法确认当前客户端对应的cacheserver的hash值以及要存储数据key的hash进行对应,确认cacheserver,获取connection进行数据存储
2、memcache的get方法:通过一致性hash算法确认当前客户端对应的cacheserver的hash值以及要提取数据的hash值,进而确认存储的cacheserver,获取connection进行数据提取
五、总结
1、一致性hash算法只是帮我们减少cache集群中的机器数量增减的时候,cache的数据能进行最少重建。只要cache集群的server数量有变化,必然产生数据命中的问题
2、对于数据的分布均衡问题,通过虚拟节点的思想来达到均衡分配。当然,我们cache server节点越少就越需要虚拟节点这个方式来均衡负载。
3、我们的cache客户端根本不会维护一个map来记录每个key存储在哪里,都是通过key的hash和cacheserver(也许ip可以作为参数)的hash计算当前的key应该存储在哪个节点上。
4、当我们的cache节点崩溃了。我们必定丢失部分cache数据,并且要根据活着的cache server和key进行新的一致性匹配计算。有可能对部分没有丢失的数据也要做重建...
5、至于正常到达数据存储节点,如何找到key对应的数据,那就是cache server本身的内部算法实现了,此处不做描述。
一、概述
1、什么是spymemcached
spymemcached是一个memcached的客户端,http://code.google.com/p/spymemcached/
它使用了一致性hash算法ketama进行数据存储节点的选择。与常规的hash算法思路不同,只是对我们要存储数据的key进行hash计算,分配到不同节点存储。一致性hash算法是对我们要存储数据的服务器进行hash计算,进而确认每个key的存储位置。
2、常规hash算法的应用以及其弊端
最常规的方式莫过于hash取模的方式。比如集群中可用机器适量为N,那么key值为K的的数据请求很简单的应该路由到hash(K) mod N对应的机器。的确,这种结构是简单的,也是实用的。但是在一些高速发展的web系统中,这样的解决方案仍有些缺陷。随着系统访问压力的增长,缓存系统不得不通过增加机器节点的方式提高集群的相应速度和数据承载量。增加机器意味着按照hash取模的方式,在增加机器节点的这一时刻,大量的缓存命不中,缓存数据需要重新建立,甚至是进行整体的缓存数据迁移,瞬间会给DB带来极高的系统负载,设置导致DB服务器宕机。
3、设计分布式cache系统时,一致性hash算法可以帮我们解决哪些问题?
分布式缓存设计核心点:在设计分布式cache系统的时候,我们需要让key的分布均衡,并且在增加cache server后,cache的迁移做到最少。
这里提到的一致性hash算法ketama的做法是:选择具体的机器节点不在只依赖需要缓存数据的key的hash本身了,而是机器节点本身也进行了hash运算。
二、一致性哈希算法情景描述
1、 hash机器节点
首先求出机器节点的hash值(怎么算机器节点的hash?ip可以作为hash的参数吧。。当然还有其他的方法了),然后将其分布到0~2^32的一个圆环上(顺时针分布)。如下图所示:
图1-1
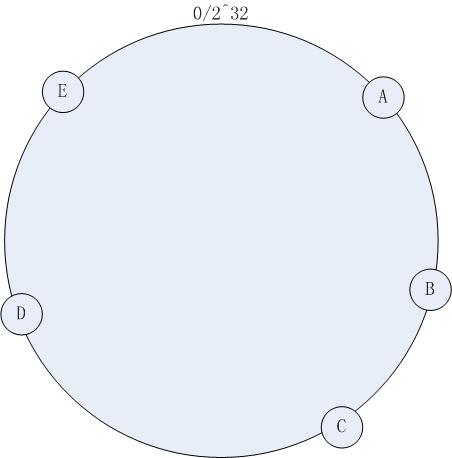
图1-2
集群中有机器:A , B, C, D, E五台机器,通过一定的hash算法我们将其分布到如上图所示的环上。
2、访问方式
如果有一个写入缓存的请求,其中Key值为K,计算器hash值Hash(K), Hash(K) 对应于图 – 1环中的某一个点,如果该点对应没有映射到具体的某一个机器节点,那么顺时针查找,直到第一次找到有映射机器的节点,该节点就是确定的目标节点,如果超过了2^32仍然找不到节点,则命中第一个机器节点。比如 Hash(K) 的值介于A~B之间,那么命中的机器节点应该是B节点(如上图 )。
3、增加节点的处理
如上图 1– 1和图1-2,在原有集群的基础上欲增加一台机器F,增加过程如下:
计算机器节点的Hash值,将机器映射到环中的一个节点,如下图:
图2-1
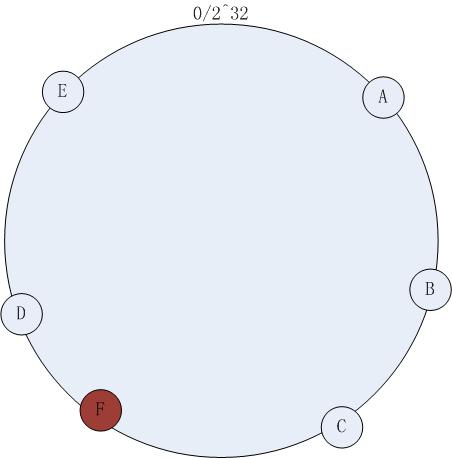
图2-2
增加机器节点F之后,访问策略不改变,依然按照(2)中的方式访问,此时缓存命不中的情况依然不可避免,不能命中的数据是hash(K)在增加节点以前落在C~F之间的数据。尽管依然存在节点增加带来的命中问题,但是比较传统的 hash取模的方式,一致性hash已经将不命中的数据降到了最低。
Consistent Hashing最大限度地抑制了hash键的重新分布。另外要取得比较好的负载均衡的效果,往往在服务器数量比较少的时候需要增加虚拟节点来保证服务器能均匀的分布在圆环上。因为使用一般的hash方法,服务器的映射地点的分布非常不均匀。使用虚拟节点的思想,为每个物理节点(服务器)在圆上分配100~200个点。这样就能抑制分布不均匀,最大限度地减小服务器增减时的缓存重新分布。用户数据映射在虚拟节点上,就表示用户数据真正存储位置是在该虚拟节点代表的实际物理服务器上。
下面有一个图描述了需要为每台物理服务器增加的虚拟节点。

图三
x轴表示的是需要为每台物理服务器扩展的虚拟节点倍数(scale),y轴是实际物理服务器数,可以看出,当物理服务器的数量很小时,需要更大的虚拟节点,反之则需要更少的节点,从图上可以看出,在物理服务器有10台时,差不多需要为每台服务器增加100~200个虚拟节点才能达到真正的负载均衡。
三、以spymemcache源码来演示虚拟节点应用
1、上边描述的一致性Hash算法有个潜在的问题是:
(1)、将节点hash后会不均匀地分布在环上,这样大量key在寻找节点时,会存在key命中各个节点的概率差别较大,无法实现有效的负载均衡。
(2)、如有三个节点Node1,Node2,Node3,分布在环上时三个节点挨的很近,落在环上的key寻找节点时,大量key顺时针总是分配给Node2,而其它两个节点被找到的概率都会很小。
2、这种问题的解决方案可以有:
改善Hash算法,均匀分配各节点到环上;[引文]使用虚拟节点的思想,为每个物理节点(服务器)在圆上分配100~200个点。这样就能抑制分布不均匀,最大限度地减小服务器增减时的缓存重新分布。用户数据映射在虚拟节点上,就表示用户数据真正存储位置是在该虚拟节点代表的实际物理服务器上。
在查看Spy Memcached client时,发现它采用一种称为Ketama的Hash算法,以虚拟节点的思想,解决Memcached的分布式问题。
3、源码说明
该client采用TreeMap存储所有节点,模拟一个环形的逻辑关系。在这个环中,节点之前是存在顺序关系的,所以TreeMap的key必须实现Comparator接口。
那节点是怎样放入这个环中的呢?
KetamaNodeLocator.java中
[html] view
plain copy
protected void setKetamaNodes(List<MemcachedNode> nodes) {
TreeMap<Long, MemcachedNode> newNodeMap = new TreeMap<Long, MemcachedNode>();
int numReps= config.getNodeRepetitions();
for(MemcachedNode node : nodes) {
// Ketama does some special work with md5 where it reuses chunks.
if(hashAlg == HashAlgorithm.KETAMA_HASH) {
for(int i=0; i<numReps / 4; i++) {
byte[] digest=HashAlgorithm.computeMd5(config.getKeyForNode(node, i));
for(int h=0;h<4;h++) {
Long k = ((long)(digest[3+h*4]&0xFF) << 24)
| ((long)(digest[2+h*4]&0xFF) << 16)
| ((long)(digest[1+h*4]&0xFF) << 8)
| (digest[h*4]&0xFF);
newNodeMap.put(k, node);
getLogger().debug("Adding node %s in position %d", node, k);
}
}
} else {
for(int i=0; i<numReps; i++) {
newNodeMap.put(hashAlg.hash(config.getKeyForNode(node, i)), node);
}
}
}
assert newNodeMap.size() == numReps * nodes.size();
ketamaNodes = newNodeMap;
config.getNodeRepetitions()表示的是需要为每台物理服务器扩展的虚拟节点倍数(scale)。
上面的流程大概可以这样归纳:四个虚拟结点为一组,以getKeyForNode方法得到这组虚拟节点的name,Md5编码后,每个虚拟结点对应Md5码16个字节中的4个,组成一个long型数值,做为这个虚拟结点在环中的惟一key。第10行k为什么是Long型的呢?就是因为Long型实现了Comparator接口。
默认节点配置文件如下:DefaultKetamaNodeLocatorConfiguration.java
package net.spy.memcached.util; import java.util.HashMap; import java.util.Map; import net.spy.memcached.MemcachedNode; /** * A Default implementation of the configuration required for the * KetamaNodeLocator algorithm to run. */ public class DefaultKetamaNodeLocatorConfiguration implements KetamaNodeLocatorConfiguration { private final int numReps = 160; // Internal lookup map to try to carry forward the optimisation that was // previously in KetamaNodeLocator protected Map<MemcachedNode, String> socketAddresses = new HashMap<MemcachedNode, String>(); /** * Returns the socket address of a given MemcachedNode. * * @param node The node which we're interested in * @return String the socket address of that node. */ protected String getSocketAddressForNode(MemcachedNode node) { // Using the internal map retrieve the socket addresses // for given nodes. // I'm aware that this code is inherently thread-unsafe as // I'm using a HashMap implementation of the map, but the worst // case ( I believe) is we're slightly in-efficient when // a node has never been seen before concurrently on two different // threads, so it the socketaddress will be requested multiple times! // all other cases should be as fast as possible. String result = socketAddresses.get(node); if (result == null) { result = String.valueOf(node.getSocketAddress()); if (result.startsWith("/")) { result = result.substring(1); } socketAddresses.put(node, result); } return result; } /** * Returns the number of discrete hashes that should be defined for each node * in the continuum. * * @return NUM_REPS repetitions. */ public int getNodeRepetitions() { return numReps; } /** * Returns a uniquely identifying key, suitable for hashing by the * KetamaNodeLocator algorithm. * * <p> * This default implementation uses the socket-address of the MemcachedNode * and concatenates it with a hyphen directly against the repetition number * for example a key for a particular server's first repetition may look like: * <p> * * <p> * <code>myhost/10.0.2.1-0</code> * </p> * * <p> * for the second repetition * </p> * * <p> * <code>myhost/10.0.2.1-1</code> * </p> * * <p> * for a server where reverse lookups are failing the returned keys may look * like * </p> * * <p> * <code>/10.0.2.1-0</code> and <code>/10.0.2.1-1</code> * </p> * * @param node The MemcachedNode to use to form the unique identifier * @param repetition The repetition number for the particular node in question * (0 is the first repetition) * @return The key that represents the specific repetition of the node */ public String getKeyForNode(MemcachedNode node, int repetition) { return getSocketAddressForNode(node) + "-" + repetition; } }
处理完正式结点在环上的分布后,可以开始key在环上寻找节点的游戏了。
对于每个key还是得完成上面的步骤:计算出Md5,根据Md5的字节数组,通过Kemata Hash算法得到key在这个环中的位置。
KetamaNodeLocator.java中
[html] view
plain copy
MemcachedNode getNodeForKey(long hash) {
final MemcachedNode rv;
if(!ketamaNodes.containsKey(hash)) {
// Java 1.6 adds a ceilingKey method, but I'm still stuck in 1.5
// in a lot of places, so I'm doing this myself.
SortedMap<Long, MemcachedNode> tailMap=getKetamaNodes().tailMap(hash);
if(tailMap.isEmpty()) {
hash=getKetamaNodes().firstKey();
} else {
hash=tailMap.firstKey();
}
}
rv=getKetamaNodes().get(hash);
return rv;
}
/** * @return the ketamaNodes */ protected TreeMap<Long, MemcachedNode> getKetamaNodes() { return ketamaNodes; }
上边代码的实现就是在环上顺时针查找,没找到就去的第一个,然后就知道对应的物理节点了。
四、应用场景分析
1、memcache的add方法:通过一致性hash算法确认当前客户端对应的cacheserver的hash值以及要存储数据key的hash进行对应,确认cacheserver,获取connection进行数据存储
2、memcache的get方法:通过一致性hash算法确认当前客户端对应的cacheserver的hash值以及要提取数据的hash值,进而确认存储的cacheserver,获取connection进行数据提取
五、总结
1、一致性hash算法只是帮我们减少cache集群中的机器数量增减的时候,cache的数据能进行最少重建。只要cache集群的server数量有变化,必然产生数据命中的问题
2、对于数据的分布均衡问题,通过虚拟节点的思想来达到均衡分配。当然,我们cache server节点越少就越需要虚拟节点这个方式来均衡负载。
3、我们的cache客户端根本不会维护一个map来记录每个key存储在哪里,都是通过key的hash和cacheserver(也许ip可以作为参数)的hash计算当前的key应该存储在哪个节点上。
4、当我们的cache节点崩溃了。我们必定丢失部分cache数据,并且要根据活着的cache server和key进行新的一致性匹配计算。有可能对部分没有丢失的数据也要做重建...
5、至于正常到达数据存储节点,如何找到key对应的数据,那就是cache server本身的内部算法实现了,此处不做描述。
相关文章推荐
- Memcached学习笔记
- 在Linux上安装Memcached服务
- 在ThinkPHP3.2中使用memcache
- 关于 Memcached 的一些使用
- 【转载】在Windows下Memcache缓存系统构建(Java)
- ehcache memcache redis三大缓存男高音
- memcached 的简单使用
- Linux安装Memcached
- memcache永久有效数据丢失
- 使用PHP简单操作Memcached
- Memcache 雪崩现象
- Memcache内存管理
- Memcache入门
- linux上安装memcached步骤
- Memcached 及 Redis 架构分析和比较
- mac memcache常用命令
- php+memcached配置
- c# 中使用memcached
- Memcached
- memcache 1.4.24源码分析